Vision
The grand promises of the postgenomic era are the academic, systems biologic understanding of the normal and disease states at physiological, cognitive, and emotional levels; the personalized prevention, diagnosis, drugs, and treatments of diseases significant in public health; and rational drug design in the pharma industry. However it is more and more clear that their fulfillment requires new technological breakthroughs to measure unprecedented genomic, proteomic, metabolomic, or immunomic profiles, new solutions for the representation of biomedical knowledge, new approaches to induction for statistical and causal inferences. A key factor for the integration of the myriads of biological knowledge items (mega-scale memes) and omic data (giga- and tera-scale data from multiple levels) is the efficient use of massive computational resources (tera- and peta-scale computing).
For the field of knowledge representation, knowledge engineering, and decision support, the central role of biomedicine is unchallenged from the birth of this field. However the explosion of the sheer amount of electronically available biomedical knowledge, its use in supporting the design of experiments and data collection, its transformation to a form eligible for the incorporation in inductive inference, and the fusion of the uncertain results of inductive inference with factual knowledge to support the interpretation of the data analysis pose serious new challenges.
Within the biomedical world it became evident that the understanding of the deciphered human genome requires the understanding of the effects of its evolutionary (phylogenetic), population level (germ-line), and individual (somatic) variations, besides other artificial perturbations and interventions. Today thousands of complete genomes are available. The exploration of the genetic and epigenetic germline variations has been accelerated in the last decade. Currently the complete genomic exploration of somatic mutations has started, such as in tumorgenetics and immunomics, and more and more complete and exact proteomic, lipidomic, and metabolomic profiles are available, which are more and more complex as dependent on the environment and life style. Another extension is the exploration of symbiotic organisms as well using metagenomics.
For inductive inference, the main challenges of the high-throughput era of biomedicine are the high-dimensional data sets, the small sample size, and the easy access and multiple use of many data sets, besides standard, though pressing issues such as the use of incomplete data. The most important responses are based on the incorporation of prior knowledge and the use of massive amount of computer power. Monte Carlo methods in Bayesian statistics and resampling techniques from the field of computer intensive statistics are good examples for the applied computer intensive techniques. The loss of the leading position of nuclear simulations at the field of high-performance computing was a surprise five years ago, but the pioneering role of bioinformatics is well recognised today.
Thus biomedical research is at the crossroad of knowledge engineering, decision support, inductive inference (statistics), and high-performance computation.
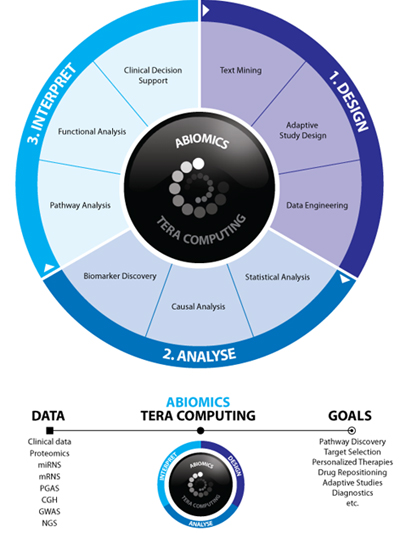
By realizing this constellation, the focus of Abiomics Europe provides tools for the complete chain of study design, data analysis, interpretation, and decision support, with particular emphases on the integration of data from heterogeneous levels and the fusion of background knowledge. Specifically we offer tools for the following:
- Sequential study design
- Biomarker analysis
- Knowledge base technologies for metaanalysis
- Clinical decision support
- Target and lead prioritization by repositioning
- Save a drug by personalization
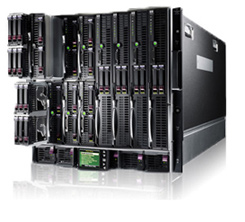