Biomarker discovery
The basic objective of biomarker search is to identify factors that indicate the presence of a particular disease or an abnormal condition. The identification of molecular biomarkers from a multitude of test compounds and components is equivalent of the feature subset selection problem (FSS) known in the field of machine learning. This can be defined as follows: given a target variable (e.g.: a disease status) and a set of explanatory variables (e.g.: clinical parameters, the results of proteomic and genomic tests, etc), the aim of feature subset selection is to identify those features (i.e. variables) that are relevant with respect to the target variable, that is the state of the target variable can be inferred from their state.The methodology we use, applies a probabilistic approach based on the structural properties of Bayesian networks. This method assigns a probability value to each individual entity, which provides a degree of relevance. An important feature of this method is that the probability value of the entity can be extended "upwards" depending on its "level" within the molecular biological system, so for example, the meaning of the relevance of a genotyped SNP can be investigated on the level of genes and the level of pathways. In this context, therefore, highly relevant entities may be considered as biomarkers.
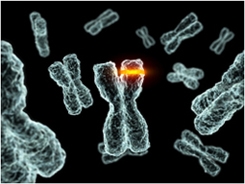