Pathway analysis
High-throughput measurement methods allow hypothesis free measurement and ensure overall “omic” view over the domain, but they typically result in limited statistical power and significance because of the limited sample number. To boost results by aggregation and integration, the analysis and interpretation of biomedical studies are more and more integrated with networks, pathways, and complex, hierarchic causal models related to systems biology.
On one hand it means more and more complex multivariate models in data analysis, ranging from simple association/covariance networks, to dependency maps and causal diagrams, and to regulatory networks with feedback loops. However the complexity of these models practically excludes the possibility of their identification, and they can be used only in model averaging frameworks, which further increase the computational burden.
On the other hand pathways are used more and more to boost and interpret the typically weak and blurred results of general data analysis of high-throughput measurements. Within this approach results are integrated according to the semantics of the pathways, for example along a causal path.
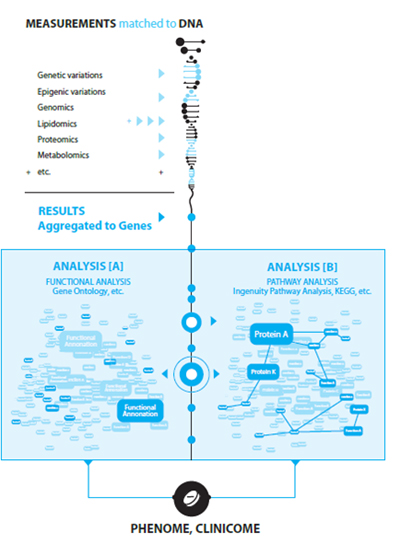
We provide tools for various network-based analysis, including dependency maps, pathways, and causal models. Using text-mining methods and manually curated, proprietary, disease specific knowledge bases we offer tools for pathway-based interpretation as well. Our integrated solution follows a unique, dual pathway-based analysis – pathway-based interpretation approach, offering a unique combination for omic data, mega-memes, and tera-computing resources.
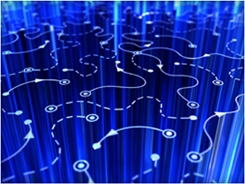