Data engineering
The quality of data analysis and decision support depends crucially on the quality of the input data. In a broad sense, data engineering starts from the selection of the abstraction level of the data, includes the management and interfacing with laboratory measurement and information systems, the design of biobanks and databases, continues with quality control, and ends with the preprocessing of the data.
Data preprocessing is typically a central issue in any real-life data analysis and decision support problem - separated from the data collection phase -, and data engineering is frequently narrowed down and interpreted as data preprocessing, involving cleaning, filtering, dimension reduction, normalization, and transformation, even imputation of the data. The richness of new biomedical measurement techniques brought an unprecedented complexity of data preprocessing methods, crucially influencing the usability of raw data coming from genetic, genomic, proteomic, lipidomic or metabolomic level.
A common element in the heterogeneous preprocessing methods is the uncertainty of the resulting data, which is further complicated by the uncertainty of starting raw data.
In our data analysis and decision support methods, the unavoidable uncertainty of the data (for example missing clinical data or noisy measurements etc.) is preserved normatively and managed by computer-intensive averaging techniques theoretically integrated with model averaging.
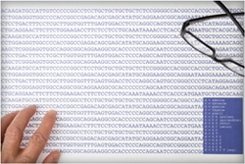